Consider it a success case or a bright point of discovery in the search for cancer inhibitors. An international team of researchers has identified a new compound to potentially disarm AKR1C3, an upregulated enzyme found in prostate, breast and other cancers, using AI-based drug screening and brilliant X-ray light at MAX IV. Is this study a diamond in the rough or a glimpse of more to come in biomedical research using artificial intelligence?
Researchers from the University of Turin in Italy and the University of Copenhagen in Denmark were interested in improvements of bioavailability of carboxylic acid groups characteristic of two NSAIDS known to inhibit AKR1C3. They sought novel chemical scaffolds the enzyme would recognize on its active site. To streamline the long process of drug discovery, the group participated in the Atomwise AIMS Awards programme, an initiative which included studies from 482 academic labs and 257 institutions across 30 countries.
The initial virtual screening conducted through the AIMS (Artificial Intelligence Molecular Screen) programme tapped AtomNet, a deep learning neural network designed for structure-based drug design and discovery. Training data for AtomNet’s AI models consists of millions of quantitative binding affinity measurements, including pKi and pIC50 values, collected from public resources like ChEMBL and PubChem as well as commercial databases such as Reaxys and Liceptor, along with proprietary sources. This data includes thousands of proteins and allows searches across chemical libraries of more than 15 quadrillion synthesizable compounds.
Drawing from a filtered subset of the Mcule library, AtomNet served 87 potential inhibitory compounds during virtual screening. The research team performed biological screening of these, and, after a battery of biochemical tests, selected four compounds for further evaluation. From this sampling, compound 4 was identified as a hit with a scaffold for AKR1C3 inhibitory activity never before reported in the literature.
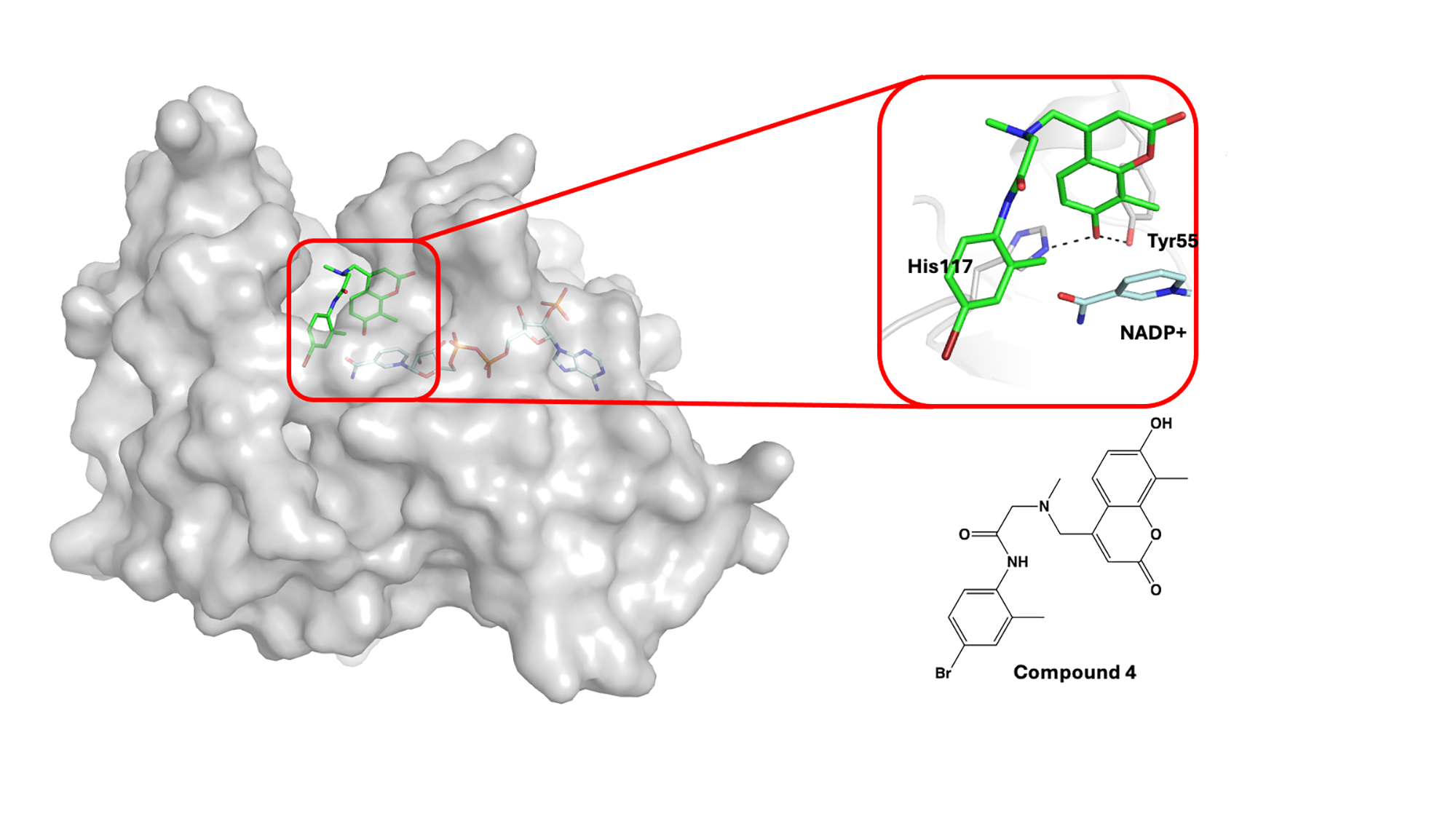
The next challenge was to understand how compound 4 interacted with AKR1C3 to inhibit its activity—knowledge that atomic-level data could enable. Using X-ray diffraction data collected at MAX IV’s BioMAX beamline, the 3D structure of AKR1C3 in complex with cofactor NADP+ and compound 4 was solved. Analysis revealed that the 7-hydroxy group (7-hydroxycoumarin scaffold) of compound 4 interacted with the oxyanion site of the enzyme. The findings could be used to develop a new series of AKR1C3 inhibitors, according to the researchers.
“One key lesson from working with AI-based screening of drug compounds is the critical importance of data quality. A representative dataset is essential for reliable results,” explained Donatella Boschi, study author and Associate Professor in Medicinal Chemistry at the University of Turin. “Additionally, AI models thrive on iterative improvement, so integrating experimental feedback into subsequent training cycles can greatly enhance their performance. However, while AI significantly accelerates drug discovery, it cannot replace traditional validation processes, human expertise in interpreting results or careful experimental design.”
The next level of biomedical research is taking shape with the advantageous combination of AI-powered prediction and high-resolution, atomic structural data.
Computational drug screening has been steadily integrated into biomedical research for the last 30 years. What differs today that enables expanded use and more effective results with AI in bioscience? According to the researchers, an extreme boost in computing power to process vast data sets, major improvements to machine learning algorithms for predicting molecular interaction and biological effects, and advances in proteomics, genomics, and screening are strong, converging factors. Add to that the human motivation of time, always of the essence.
“The primary reason for the appealing fruition of AI in the medicinal chemistry research field is the need to address the complex and time-consuming nature of traditional drug discovery methods, which often rely on labour-intensive techniques,” said Boschi. “AI, particularly machine learning, offers the potential to accelerate these processes and enhance their efficiency, making it an increasingly valuable tool in the field.”
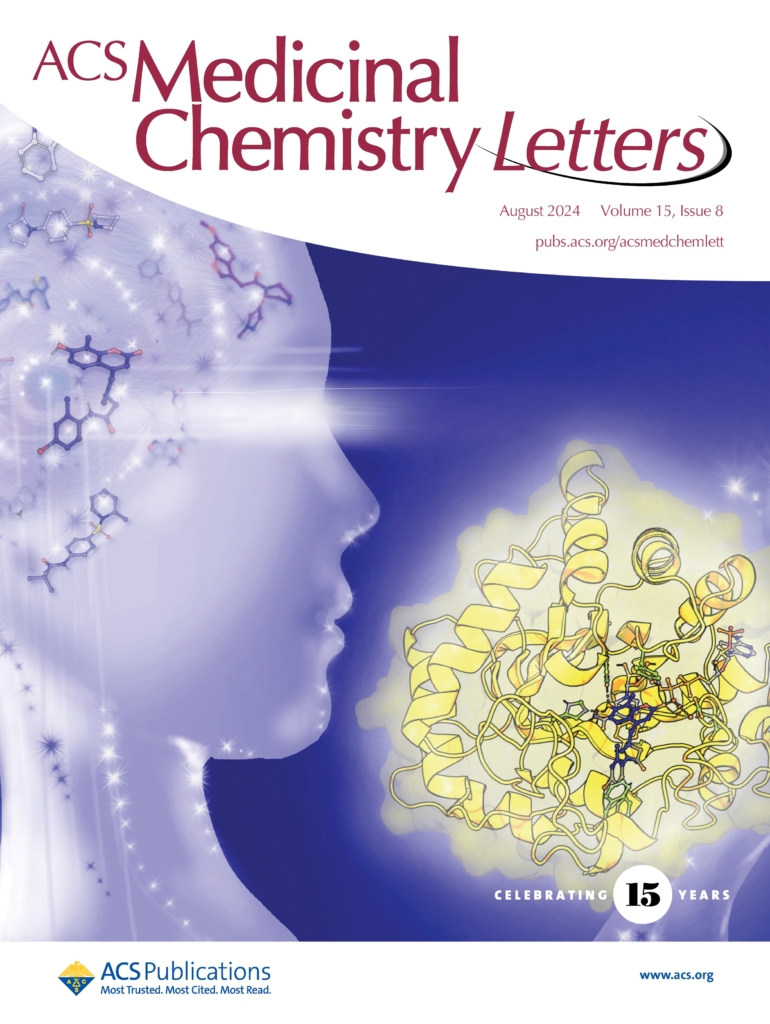
Boschi and colleagues from the University of Turin MedSynth group plan to continue the AKR1C3 research with investigations of compound 4 activity a standalone treatment and in combination with other approved first-line anti-tumour therapies.
The group’s current research focus is the design of innovative heterocyclic molecules targeting various oncological conditions, particularly acute myeloid leukemia (AML), prostate cancer, and rare tumours as well as neglected diseases such as malaria and tuberculosis.
Accelerate with AI, validate with X-rays
Since 2020 following the AIMS initiative, the University of Turin has continued collaboration with Atomwise, the California-based pharmaceutical company that uses artificial intelligence and machine learning for small molecule drug discovery. In fact, the options for AI research tools continue to expand for biomedical studies. Data prediction using computational methods, known as in silico prediction, has been gaining traction in research communities and accelerated substantially in the 2010s with the rise in AI technologies and computing power.
AI-enhanced in silico predictions can filter biochemical data, which in turn enables time and cost savings, better planning for studies, and can augment experimental testing in vivo and in vitro. Autonomous experimentation (AE) systems are another evolving area to enable human-AI collaboration solutions such as AI-driven virtual laboratories and instrument robotics.
Scientists today can enhance research results with complementary techniques using brilliant X-ray and neutrons for atomic level data. The next level of biomedical research is taking shape with the advantageous combination of high-resolution structural data from lightsources and AI-powered platforms such as AtomNet, AlphaFold, which researchers use to predict protein structure, or even the upcoming AlphaFold3 with reported capabilities for protein, RNA, DNA, and ligand model interactions.